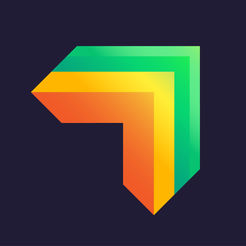
Raiz Invest partners with UNSW to power rewards with machine learning
Raiz Invest Limited, the mobile-first micro-investing platform, has partnered with UNSW and with support from the NSW Government to develop a machine-learning recommendation engine for its Raiz Rewards.
The new technology will recommend to Raiz customers more relevant offers and brands. It’s designed to provide a more seamless, personalised experience for customers when navigating more than 165 brands on Raiz Rewards.
With a customer base of more than 179,000, skewing towards the under-35 demographic, the intuitive experience provided by machine-learning will better address the demands of this millennial audience.
“Millennials have grown up with technology at their fingertips. They expect all their interactions to be easy to navigate and, above all, customised to their needs,” Raiz Invest Managing Director, George Lucas, says. “We had this customer in mind when partnering with UNSW to bring machine-learning to the Raiz platform. We’re passionate about finding new and innovative ways to add value to everyday lives. Helping customers find the right rewards for their needs is a key part of this.”
Greater personalisation and relevance
The machine-learning engine powering the Raiz Rewards platform was designed and built in collaboration with UNSW’s Dr Lina Yao, a leading machine-learning researcher with extensive experience in recommender systems. Raiz was awarded a matching $15,000 TechVouchers research grant through the NSW Government’s Boosting Business Innovation Program to work with UNSW on the project.
Deputy Premier and Minister for Small Business John Barilaro said through the ‘Boost’ program the NSW Government has provided $18 million to the state’s 11 universities and the CSIRO to work with businesses to create great new ideas and products.
“This is about entrepreneurs tapping into the top-notch research provided by our university sector,” Mr Barilaro said.
“As a government we want to do everything we can to create the right environment for people to have the confidence to launch a startup and then get the support they need to succeed. As a result, NSW is now home to nearly 49 per cent of Australia’s startups.”
What has been developed by Raiz and UNSW combines two common approaches, used by companies such as Amazon or Netflix, to a recommendation engine to give a higher level of personalisation and relevance. For example, most retailers can provide recommendations using customer specific information, “who bought this product also bought…”, or using item data, “This item is purchased with…”. The new recommendation engine blends these two approaches.
This was achieved by constructing two parallel neural networks, where the predictions from each were weighted and summed up, to provide a final prediction. The resulting model should give more relevant recommendations, without needing to incorporate complex information about either the user or the product.
“By combining these user-based and item-based frameworks, the platform is able to better define what we mean by the right product and the right customer,” Lucas says. “It’s exciting from a technical perspective but even better for customers. They will receive a higher level of personalisation and more relevant recommendations when using our platform.”
“It is an amazing experience for me and my PhD student, Shuai Zhang, working with Raiz on this project,” said Senior UNSW Lecturer, Dr Lina Yao. “We designed a new neural network architecture and structural neural recommender (SNR) for effective recommendation which leverages multi-faceted structural information hidden from varied data streams. We’re excited to see how the new techniques developed from this collaboration can contribute to Raiz’ end-user experience. I look forward to seeing our collaborations go from strength to strength.”
Raiz Invest and UNSW are continuing their technology partnership with more exciting developments in the works. These include enhancements to the recommender system and improved cybersecurity.