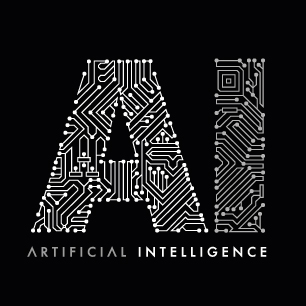
Rich Data Corp presents research findings at prestigious artificial intelligence conference
It is a win-win for both financial institutions and modern-day borrowers when it comes to using advanced artificial intelligence (AI) techniques to assess non-traditional credit histories and drive responsible lending solutions a recent study by Rich Data Corp has found.
The global credit risk company is presenting the findings from its research paper, ‘Building a Credit Risk Model using Transfer Learning and Domain Adaptation’ at AAAI this week – an annual conference dedicated to significant research into artificial intelligence.
Transfer learning and domain adaptation, the applying of algorithms from source domains and the storing of knowledge gained while solving that problem and then applying them to a different but related domains and problems that have limited data distributions, are commonly used in image and natural language processing.
The use of advanced AI techniques, transfer learning and domain adaptation can create more accurate and ethical credit risk models the research finds – and if implemented correctly, provides increased access to credit for consumers without traditional credit history that meets their financial needs and propensity to pay.
By evolving current credit risk models and increasing credit access, financial institutions could potentially see substantial economic return – even if credit score accuracy was only marginally increased.
Rich Data Corp’s Chief Product Officer, Gordon Campbell says that while the theory of transfer learning and domain adaptation is complicated, it has the potential to align traditional credit risk models with societal advancements, without reinventing the wheel.
“The world is evolving at a rapid rate and the finance sector is often hamstrung by legacy technology platforms that don’t have the flexibility to keep up. This has resulted in 58 per cent of millennials and Gen Z’s being denied at least one financial credit product along with small business owners who continue to struggle to access loans,” he said.
“Applying techniques to different sets of data in financial services has proved challenging until now. For example, BNPL providers’ predictive analytics only works for small loan amounts over short time frames, and cannot be applied for larger loans over longer periods.
“Transfer learning can be the bridge linking alternative lending data and traditional credit history assessment, but the difference in features between source and target domains, such as a source domain for a small short-term alternative loan, but the target domain may be for large and long-term instalment loan, has been challenging. By using transfer learning and domain adaptation we can more accurately combine and assess multiple sets of data that is required in credit assessments.
“For example, transfer learning and domain adaptation can combine an applicant’s short term loan data such as buy now pay later data, with their larger and longer, loan data such as a mortgage and assess more accurately that applicant’s propensity to repay a new loan.”
Rich Data Corp is building credit risk model using progressive shifting contribution networks that combine source and target domain features learning to improve model accuracy, and is adapting the features before transfer learning to get more accurate models.
Also key to this is making the explainable and transparent and showing the rules of extraction to meet compliance and responsible leading protocols.
This will also allow lenders to create new products without the need for a current customer base, and allow people with non-traditional credit histories to access credit that meets their financial needs and propensity to pay.
“Another example is an applicant that participates in the ‘gig economy’ and works four casual jobs instead of one fulltime. This applicant would not fit a traditional credit risk model, but by using transfer learning and domain adaptation, we can combine the different data sets and once again be able to more accurately understand that applicant’s propensity to repay a loan.”
Rich Data Corp will present the full findings from its research paper, ‘Building a Credit Risk Model using Transfer Learning and Domain Adaptation’ at the AAAI Conference to Artificial Intelligence.