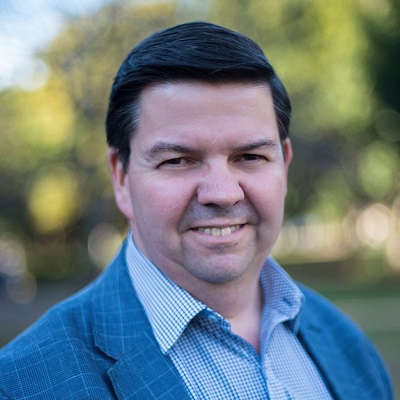
More data and improved insight will unlock the future of responsible lending
By Gordon Campbell, Chief Product Officer and Head of Strategy, Rich Data Corporation
While there has been a lot of recent noise about the possible removal of obligations outlined in ASIC’s responsible lending conduct guide (RG209) by May next year, those at the forefront of credit provision know it is unlikely to have a major bearing on the future of responsible lending. This is due to three underlying facts that govern the lending landscape and customer behaviour: the quantity and type of customer data currently available; the dramatic evolution of technology and techniques to derive data-driven insights; and the guidelines that trail the responsible use of this data and supporting techniques.
While RG209 made important first steps toward a principles-based approach to responsible lending, it didn’t address the key challenge for lenders these days: how to harness and use data accurately to lend more appropriately, how to responsibly win market share from competitors, and, at the same time, increase financial inclusion and global access to credit. And this is a Consumer and SME problem to solve.
Yes, the possible removal of RG209 may dilute the regulatory risk for lenders in relation to potential fines for misconduct. But it will not change the underlying credit risk decision and management challenges lenders now face. Under proposed changes, lenders would still need to take reasonable steps to verify a customer’s income and ability to repay. To do this well, lenders need to tailor internal processes to the needs and capabilities of their customers. Indeed, this alone would appear to justify the more extensive use of new and alternative data, and engaging more deeply with customers.
However, this is more challenging than ever as the way consumers and small businesses access credit is evolving due to the proliferation of alternative short-term lending options, such as buy-now-pay-later and innovation in merchant cashflow lending. Traditional credit risk solutions that capture and analyse historic expenses alone are no longer a great predictor of credit risk. For example, the percentage of Generation Y and Z consumers with credit cards is much lower than baby boomers or Gen Xers. Small businesses don’t borrow money against their houses as much anymore – they access things like debtor financing. These younger consumers and the current generation of start-ups do not fit traditional, outdated credit risk profiles which tend to lock them out of gaining responsible access to credit when they need it.
Smart lenders realise that the new battleground isn’t around meeting basic regulatory compliance, but better utilisation of data sources. The amount of data and information available about borrowers and the ability to collect and use it continues to evolve as it increases exponentially. Banks will still feel obliged to use that information. Perhaps not to avoid the big stick that is currently wielded by ASIC, but to reap the benefits of customer experience and positive brand recognition that come as a result of seeing customers as individuals and managing the origination experience to best meet their specific needs.
Vast pools of data about borrower activity is all well and good, however, capturing, analysing, understanding and harnessing it to help borrowers is key. And the most efficient way for lenders to do this will be through the utilisation of self-describing AI and predictive machine learning models that understand and make sense of traditional and alternative data sources to provide more accurate, transparent and compliant credit decisions. With the right systems in place, lenders can leverage technology innovation and data to service consumers with a thin credit record – or no credit history.
The real key is to deliver decisioning software that enables a lender’s Credit Risk team to evidence responsible lending outcomes. Both the model and the individual decisions must be transparent, explainable and auditable.
Importantly, responsible lending frameworks and credit risk models need to be designed to learn and adapt as new data sets emerge and understanding of borrower behaviour evolves. Lenders can safely leverage various machine learning techniques to incorporate real-world data, optimise lending outcomes and differentiate themselves. For example, predictions about future spending patterns based on historical data can be improved as real-world spending patterns are monitored, analysed and fed back into analytical models.
AI in the monitoring of borrower behavioural patterns and account conduct can also alert lenders to early hardship indicators, providing timely intervention and customer support. Lenders can also utilise loan monitoring systems to identify growth opportunities for individuals and businesses.
Incremental change to existing responsible lending processes with a one-size-fits-all approach will not meet the needs of diverse customers, nor unlock opportunities that emerging data and technology can provide in the evolving lending landscape.
By embedding responsible lending principles into their DNA, and leveraging AI innovations and emerging data, lenders can realise opportunities beyond compliance, such as improved customer satisfaction, stickiness and brand reputation. And avoid the kind of reputational damage that came about as part of the Hayne review.