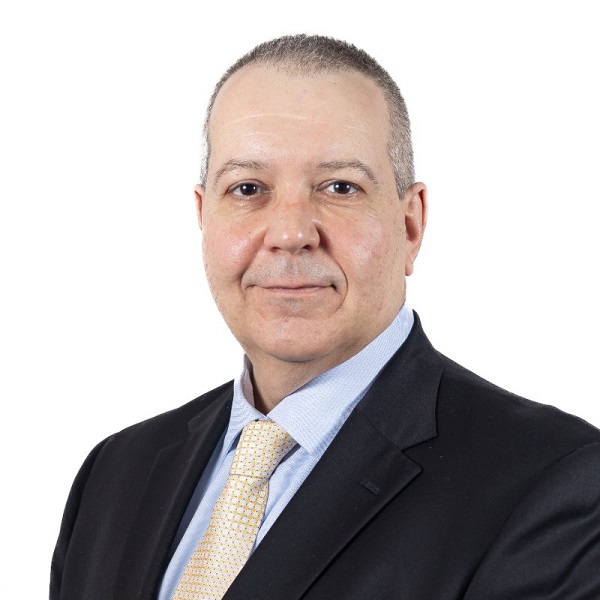
AI and Digital Transformation for Financial Services
In the rapidly evolving landscape of financial technology, discussions about Artificial Intelligence (AI) and its transformative potential have become ubiquitous. As industry leaders and innovators discuss how AI will redefine financial services, from customer experience enhancements to operational efficiencies and beyond, there’s another technological revolution quietly making its mark—low code technology. Amidst this backdrop of technological convergence, Australian FinTech sat down with financial service industry expert John Trapani (pictured), Global Industry Leader – Financial Services at Appian Corporation.
How is digital transformation shaping the financial services industry today, and what trends should organisations be aware of?
A lot of financial services firms have become collectors of technology over the years. For instance, they might have bought a piece of X to solve Y or a piece of Z to solve A. And now because platforms are capable of delivering so much within their own walls of capability, there’s a rationalisation that’s taking place now where firms are trying to say, “I actually don’t need to have 45 different tools and technologies on my IT budget. I can probably whittle that down to the handful that let me deliver all the value across all the domains that I need to worry about.”
Today there are platforms that can help organisations achieve remarkable transformations at a pace that would’ve been difficult even five years ago. A key focus for financial services organisations is in identifying those that can really help transform their entire organisation.
Low-code platforms are becoming increasingly popular for rapid application development. Can you explain how a low-code approach is changing the way financial institutions develop and deploy applications?
The standout difference between legacy approaches and modern low-code development is how low-code lets the team focus on solving business problems.
I spent the majority of my career being on and leading software development teams and until I became an Appian customer a few years ago, that meant exclusively doing it the hard way using java.net, C++, etc. And that was fine, but what it wasn’t was efficient or compatible with the needs of my end users. It was a challenge to keep SMEs and stakeholders well informed about what we were doing and how we were doing it, because we would first have to negotiate the language to capture what they needed from us and then go off and do something very technical and then come back to them and say, “is this right?”
Low code turns that on its head. There’s a collaboration that can exist between SMEs and delivery teams because you’re able to look at and work through the same visual representations of what you’re trying to solve for. And unlike in the legacy approach, the delivery teams don’t have to worry about things like solving integration challenges because the platforms are doing that for them.
So just from a collaboration standpoint, teams are much more efficient now. Business gets answers far more quickly than they did in the past. And ultimately what that means is that everyone can increasingly focus on capturing business value very, very rapidly and very iteratively.
Artificial Intelligence is attracting a lot of industry attention today, but is this a key driver of innovation in financial services? Can you outline the challenges AI technologies present to financial institutions?
Today there’s no question that generative AI is poised to drive real innovation, but it’s important to remember that we’re at a phase of the hype cycle where you really have to be mindful of the caveats.
And one of those is how to make these generative AI models produce output that’s repeatable and explainable. If you’re trying to build something for any organisation, but especially a financial institution, you need a lot of confidence that the answers you’re going to get are going to be explainable, repeatable, coherent and also safe. And I don’t quite think we’re there yet with generative AI models.
There’s also the challenges around if the model you’re using is free of copyright infringement and if you’re within your rights to use the model to run your business. I think the courts in various jurisdictions around the world are still working through those issues.
And then you must consider if it is safe. Is it going to leak the data that you’re providing? If you send in a bunch of personal information in order to make a credit decision, let’s say, do you have to worry about that data somehow being somehow slipping out of the model and being available for others to read and use? As the space matures teams will become adept at managing these risks. I think once this begins to happen, there’s going to be a rapid explosion of capability being deployed.
The example everyone either talks about or thinks of when they hear about generative AI today is customer interactions. Like what today are chat bots, it’s not hard to know you’re talking with a machine. I think in a few years you will not be able to know whether or not there’s a human on the other end of that interaction or generative AI model. Now whether that’s good or bad is a philosophical discussion that we can have some other time, but I do think there are opportunities to make the customer experience a lot smoother because you can apply gen AI once it’s ready at a scale that’s difficult to do with humans.
With stringent regulatory requirements in the finance sector, how can process automation, AI and low-code ensure that fintech’s processes are compliant and secure?
Process automation, which largely can be about taking human steps out of the loop and trying to enforce repeatable standards, is a great way to help ensure that your first line of defense is operating within acceptable parameters.
Every activity that requires people to do something can be viewed as an opportunity for error. So, if you rely on automation where you can, that’s a good way to remove some of that risk from your landscape.
So traditionally there have been these detection engines that exist and AI is used increasingly to find more subtle occurrences of transaction styles that might be evidence of crime or fraud. Over time those mechanisms and their application of AI is going to continue to grow so that we’re going to see more robust detection mechanisms, and over time, I’d expect it to become even more difficult to introduce very subtle types of criminal activity because the tools are going to get better at catching them.
Looking forward, what do you see as the future role of AI, low-code and process automation in the financial services industry over the next decade?
A lot of operations inside of financial organisations are beholden to two things. One is checklists. Many organisations live and die by their checklists, and these are sometimes hourly, but they’re certainly daily, weekly, monthly, quarterly checklists of some task needs to happen.
The other is the notion of ‘four eyes’ or ‘maker-checker’ work. So, somebody is the maker who does some task and then someone else is the checker who makes sure it’s done correctly.
And there’s an awful lot of risk mitigation today that’s based on these two things.
I think as automation tools improve, and that’s everything from the process automation capabilities that exist today combined with low code and AI, we’re going to see a transition to the ‘maker’ is the AI or the platform and only the ‘checker’ is human. So rather than have two people operating on the same task, you’re going to see a lot of the actual tasks being performed by machine and then the checker is still going to be a person.
It will end up having a net positive effect because you can rely on machines to do low value work, then upskill and train people so that they are not only better able to help train, manage and oversee the work machines are doing, but inevitably do higher value work.