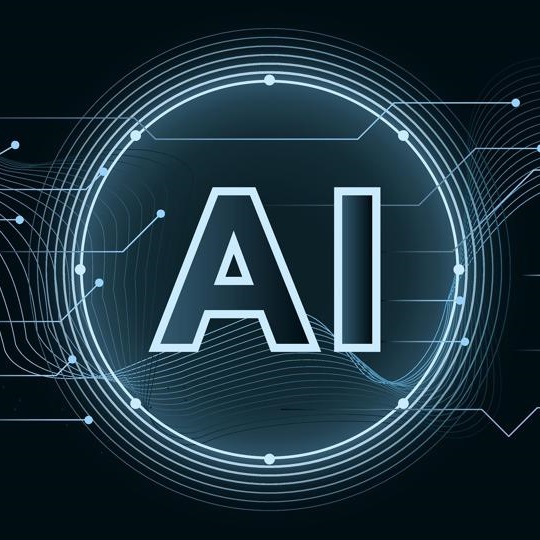
Banks made a big AI bet, now it is time to deliver
By Jonathan Tanner, Industry Principal, Financial Services APAC, Pegasystems
Over the past few years, we’ve watched as Artificial Intelligence (AI) has gone mainstream, particularly with the advent of generative and now increasingly agentic systems. The net result is that this has climbed to the top of every boardroom agenda, dominate headlines, and spur a race among financial institutions to experiment, implement, and prove value. Early adoption and pilot programs became badges of innovation as banks signal future-readiness. While early enthusiasm carried AI through the hype cycle, we’re now entering a more sobering phase, one where excitement alone won’t sustain support.
This marks a turning point for AI in banking. Boards and investors have placed their trust in CIOs, CTOs, and IT leaders to make the right bets, and if those bets don’t start showing results soon, banks may begin to reassess or reduce their AI investment strategies prematurely – a move Forrester warns could stifle long-term innovation.
The year of ROI
While interest in AI remains high, the focus is changing from experimentation to evaluation, as banks reassess what meaningful success looks like. Rather than being swayed by novelty, banks are returning to fundamentals and reassessing AI against traditional performance indicators. ROI, cost-to-serve, and customer outcomes are now the benchmarks of success, not proofs of concept or potential.
This isn’t surprising as the financial services is a sector governed by results. Banks especially are under pressure to reduce cost, manage risk, and win customer loyalty in a competitive environment. If AI is to play a role in that, it needs to be embedded where it counts such as the decisioning engines that drive customer experiences, fraud detection, credit scoring, compliance monitoring and more.
That means fewer standalone AI projects, and more strategic deployments that sit within core systems. The more AI can drive value at scale across key workflows, the more defensible the investment becomes.
Where AI has fallen short
That’s not to say the technology has failed. In fact, the core capabilities of AI, pattern recognition, predictive modelling, and intelligent automation, are more advanced than ever. What’s failed is how it’s been approached. In Australia alone, 68% of organisations have experienced a failed AI implementation, according to Pega research.
Too many organisations chased innovation without a clear problem to solve, leading to scattered initiatives that promised transformation but delivered very little. It has not uncommon to see banks with multiple generative AI pilots running simultaneously, often in isolation from each other, and unable to scale meaningfully. That fragmentation has created internal fatigue, external scepticism, and a growing disconnect between investment and value.
The technology also doesn’t thrive in a vacuum. AI without access to clean, connected data is like a jet engine without fuel. It may look powerful on paper, but it won’t get you off the ground. Add to this the complexity of legacy infrastructure, regulatory uncertainty, and cultural resistance to change, and it’s no wonder many banks are struggling to break out of the AI hype cycle and into a phase of actual returns.
The short-term vs long-term conundrum
While businesses naturally seek quick wins, AI often requires a longer runway. Developing accurate models, securing quality data, and integrating with existing infrastructure takes time. Some of the most effective AI deployments in banks have been built quietly over years – not months.
Balancing short-term wins with long-term strategy requires a disciplined approach. Leaders need to define what success looks like upfront. Is it faster loan approvals? Fewer false positives in fraud detection? More accurate customer segmentation? Once defined, teams can work to design use cases that are measurable and meaningful.
AI is only as effective as the strategy behind it
A clear, coherent AI strategy is what separates early promise from enduring performance. It begins with identifying the right business problems to solve – not those that are the most novel, but those that are the most valuable. From there, it’s about selecting use cases that are achievable with current capabilities, aligning teams across business, IT and risk, and defining what success looks like from the outset.
Crucially, a strategy must be designed to scale. That means building on connected data, embedding AI within core systems and workflows, and establishing governance frameworks to manage risk, bias, and accountability. This is particularly important in banking, where decisions must be explainable and compliant, not just fast.
An effective AI strategy also understands its limitations, but also recognises the importance of bringing together predictive, adaptive and generative AI alongside business rules as a coherent platform strategy rather than a set of bolt on capabilities. AI can accelerate decision-making, surface insights, and automate tasks, but it lacks judgment. Human oversight remains critical. The banks that succeed will be those that use AI to enhance, not replace, human expertise. Equally important is knowing when not to proceed. Not every process benefits from AI. Not every insight needs a model and avoiding overreach is as important as innovation itself.
The temptation to cut losses when ROI is unclear is understandable, but rarely beneficial. Done right, AI can deliver material impact and with all things considered, the AI bet is still a sound one, but only if it is guided by strategy, measured by outcomes, and executed with intent.
Ultimately, 2025 will be remembered not for how many AI initiatives were launched, but for how many delivered real value.